News
How to Use Statistics for Player Recruitment
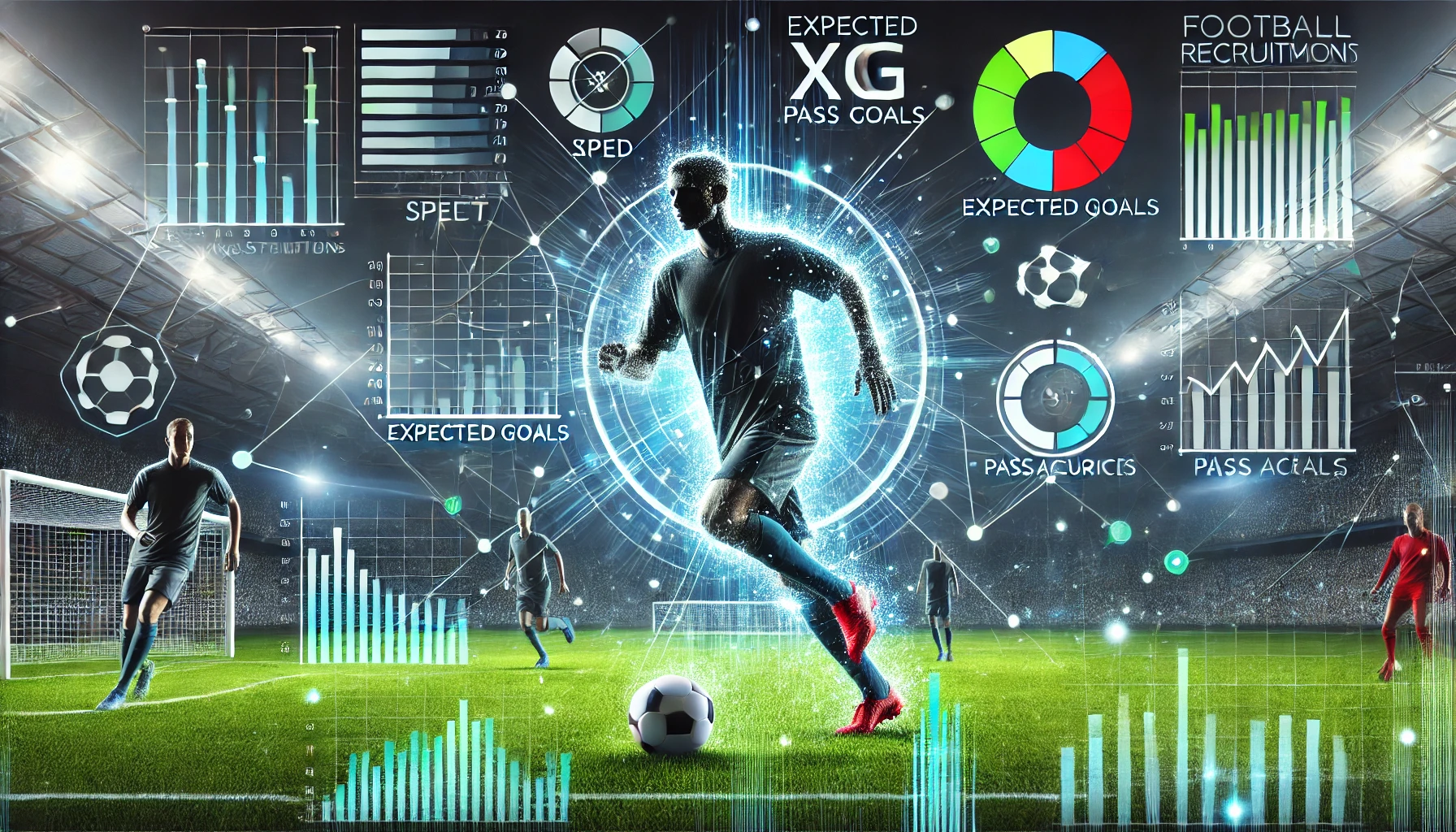
Football has experienced a significant technological shift in recent years, particularly in the way clubs utilize data analytics.
Gone are the days when clubs depended solely on scouts' intuition and traditional methods. Advanced statistical tools now play a critical role in guiding decisions on player recruitment. This shift has transformed football into a more scientific endeavor, characterized by meticulous planning and precision.
This article delves into how data analytics is shaping the future of player recruitment in football. Beyond clubs, free bets and betting enthusiasts also benefit from the growing reliance on data analytics, as more statistics provide deeper insights into player performance and match outcomes.
The Rise of Data Analytics in Football
The integration of data analytics into football began gaining traction in the early 2000s but was propelled forward by the success of Michael Lewis's book Moneyball. The book illustrated how the Oakland Athletics used data-driven strategies to revolutionize baseball. Inspired by this, football clubs, especially those in the Premier League, started exploring how analytics could enhance team performance and player acquisitions.
Pioneering clubs like Liverpool, Manchester City, and Brentford embraced this approach by assembling teams of statisticians, data scientists, and analytics experts to delve into the numbers. One key reason for the growing adoption of analytics in recruitment is its ability to reduce risks. While traditional scouting relies heavily on subjective observations, which can introduce bias, analytics provide objective measures of player performance, physical attributes, and future potential.
Key Metrics in Data-Driven Recruitment
Modern recruitment focuses on much more than just goals and assists. Advanced statistics evaluate a player's overall impact through metrics such as:
Expected Goals (xG):
This model estimates the likelihood of a shot resulting in a goal based on variables like distance, angle, and type of assist. xG helps clubs assess a player's scoring ability and dependency on chance quality.Pass Completion Percentage:
An important metric for assessing how well players perform under pressure, particularly midfielders responsible for advancing the ball.Progressive Passes and Carries:
These measure a player's ability to move the ball forward effectively through passing or dribbling, key attributes for midfielders and defenders.Defensive Actions:
Metrics such as interceptions, successful tackles, and pressuring opponents are crucial for evaluating defensive players.Physical Data:
Data on factors like distance covered, sprints per game, and top speeds helps gauge whether a player can meet the physical demands of elite football.Player Value Estimation:
Analytics are used to predict a player's future potential, which is especially useful when scouting young talent with long-term value.
Real-Life Examples of Clubs Using Data Analytics
Liverpool FC:
Under Jürgen Klopp and Michael Edwards, Liverpool used data analytics to identify undervalued players like Mohamed Salah, Sadio Mané, and Andrew Robertson. This approach turned them into a title-winning side.
Brentford FC:
Brentford relies almost entirely on data to scout undervalued players across Europe. Their strategy led to promotion to the Premier League in 2021.
Manchester City:
Combining analytics with traditional scouting, Manchester City uses extensive metrics to ensure new signings align with Pep Gladiola's system. Players like Rodri and Ruben Díaz were chosen for their passing ability, tactical awareness, and fit within City's high defensive line.
Advantages and Challenges of Data-Driven Recruitment
The use of analytics in football recruitment offers numerous benefits, including improved decision-making and reduced risks in high-value transfers. However, while data provides invaluable insights, its effectiveness depends on proper interpretation and integration with traditional methods.